Lars Ankile
I'm a visiting researcher at the Improbable AI Lab at MIT CSAIL working on robot learning with Prof. Pulkit Agrawal.
Before this, I completed an M.Eng. of Data Science at Harvard University, where I completed my thesis work
in the
Improbable AI group, working on sample-efficient imitation
learning.
I also spent a year in the Data to Actionable Knowledge Lab at
Harvard,
working with Profs. Weiwei Pan and
Finale Doshi-Velez on applying RL and
Bayesian inference to
model human decision-making for frictionful tasks in
healthcare settings.
I also spent a summer and fall interning with Prof. David
Parkes
and Matheus Ferreira in the
EconCS Lab at Harvard working on
detecting manipulation in multi-agent settings.
I did my undergrad at the Norwegian University of Science and Technology (NTNU) and did my thesis work on
applying
Deep Learning to econometric forecasting of complex and multivariate time series, supervised by
Prof. Sjur Westgaard.
Formal Bio Github G. Scholar LinkedIn Twitter Resume
lars.ankile@gmail.com / ankile@mit.edu
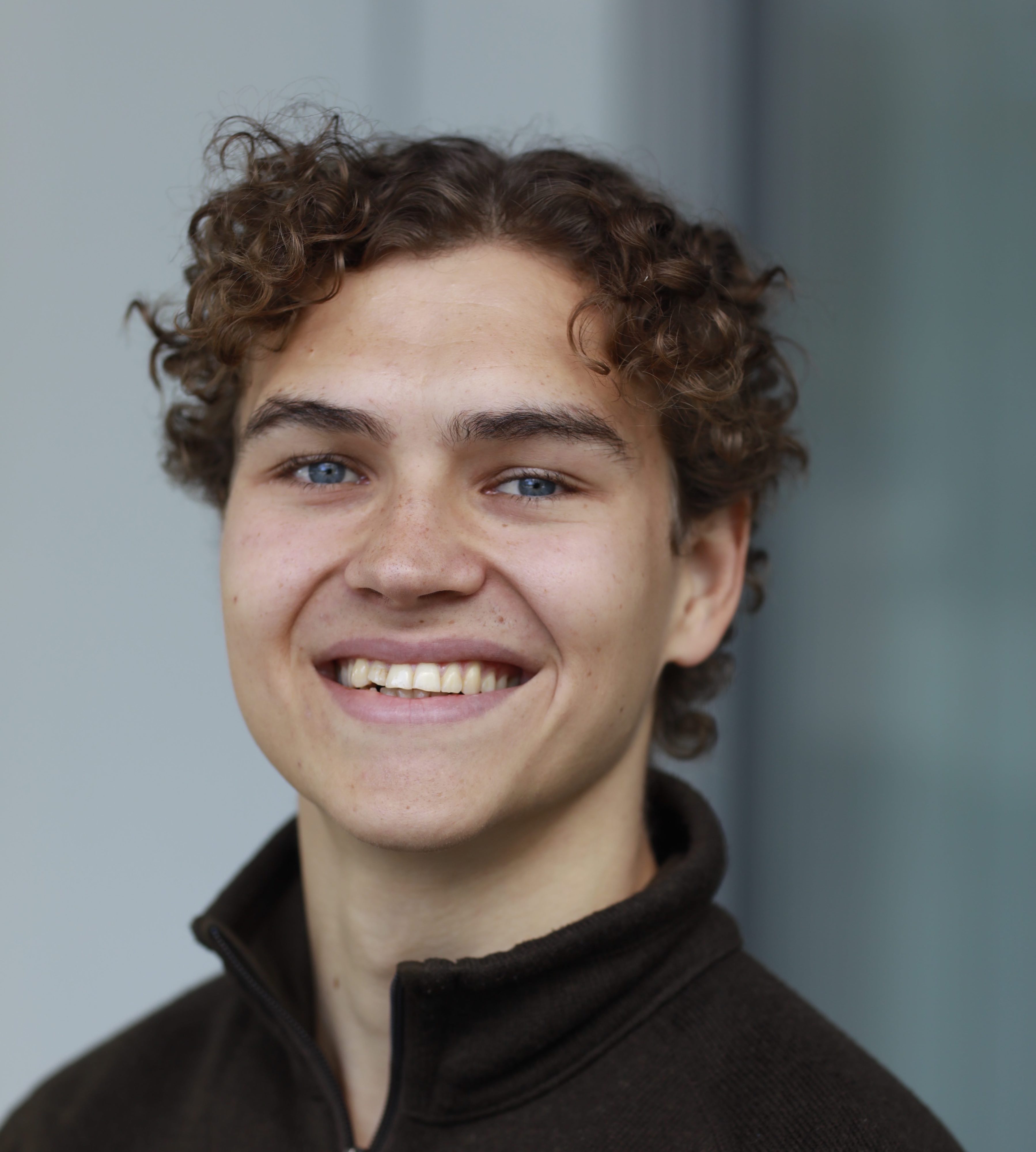
2023
Conference on Robot Learning (CoRL) Best Student Paper Award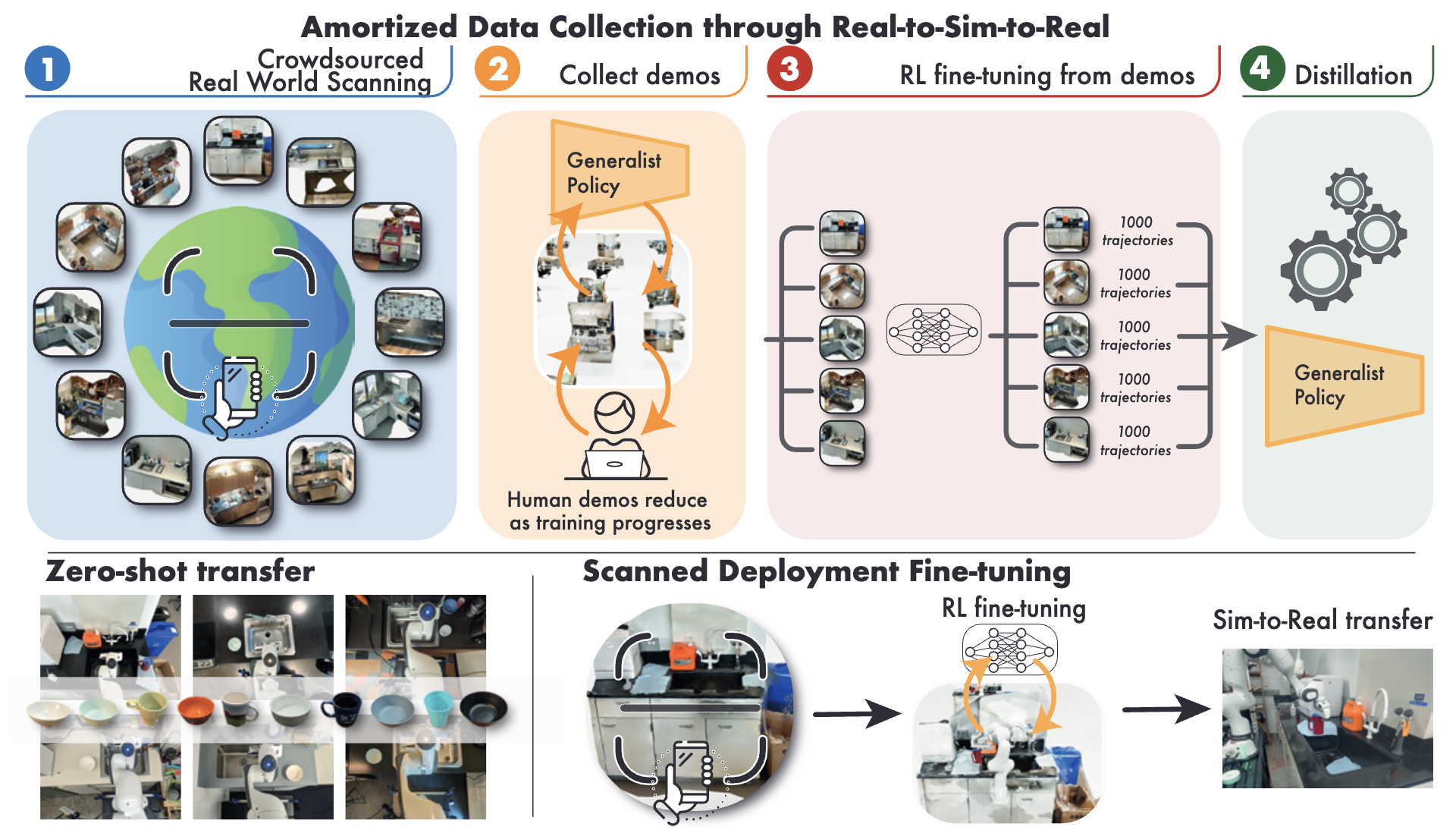
Robot Learning with Super-Linear Scaling
Marcel Torne, Arhan Jain, Jiayi Yuan, Vidaaranya Macha, Lars L Ankile, Anthony Simeonov,
Pulkit Agrawal, Abhishek Gupta
Under review
Webpage •
PDF
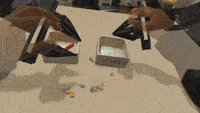
DexHub and DART: Towards Internet Scale Robot Data Collection
Younghyo Park, Jagdeep Singh Bhatia, Lars L Ankile, Pulkit Agrawal
ICRA'25
Webpage •
PDF
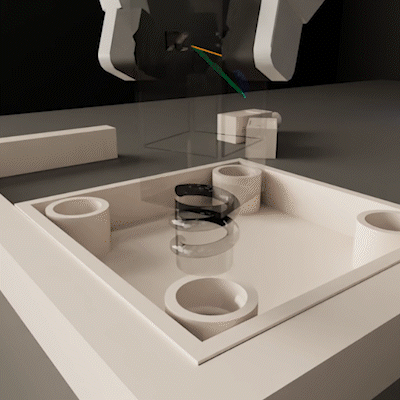
From Imitation to Refinement--Residual RL for Precise Visual Assembly
Lars L Ankile, Anthony Simeonov, Idan Shenfeld, Marcel Torne, Pulkit Agrawal
ICRA'25
Webpage •
PDF •
Code
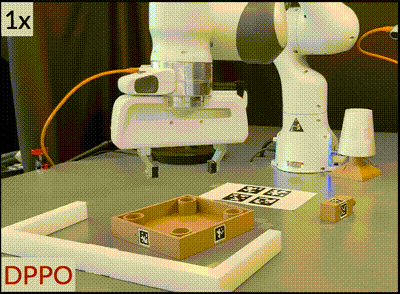
Diffusion Policy Policy Optimization
Allen Z Ren, Justin Lidard, Lars L Ankile, Anthony Simeonov, Pulkit Agrawal, Anirudha
Majumdar, Benjamin Burchfiel, Hongkai Dai, Max Simchowitz
ICLR'25
Webpage •
PDF •
Code
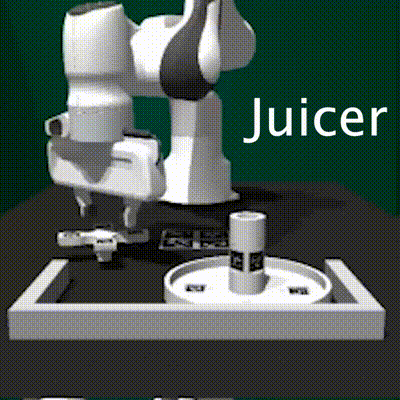
JUICER: Data-Efficient Imitation Learning for Robotic Assembly
Lars L Ankile, Anthony Simeonov, Idan Shenfeld, Pulkit Agrawal
IROS'24
Webpage •
PDF •
Code
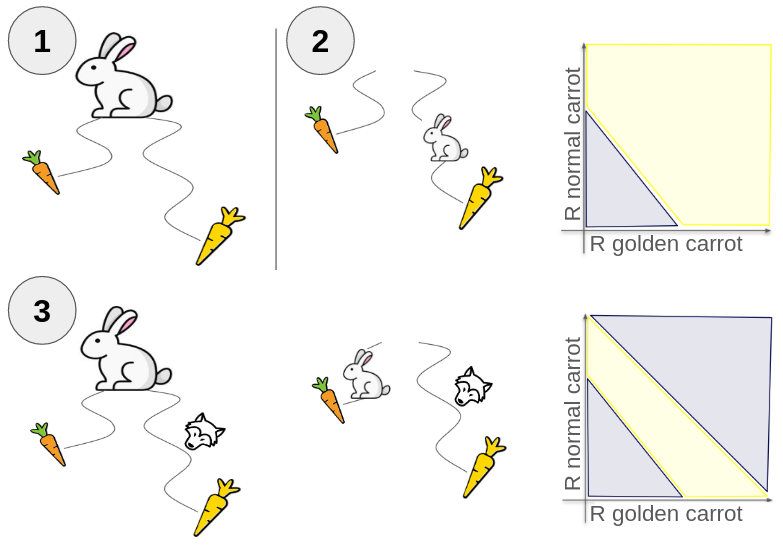
AMBER: An Entropy Maximizing Environment Design Algorithm for Inverse Reinforcement Learning
Paul Nitschke, Lars L Ankile, Eura Nofshin, Siddharth Swaroop, Finale Doshi-Velez, Weiwei
Pan
ICML'24 Workshop on Models of Human Feedback for AI Alignment
PDF
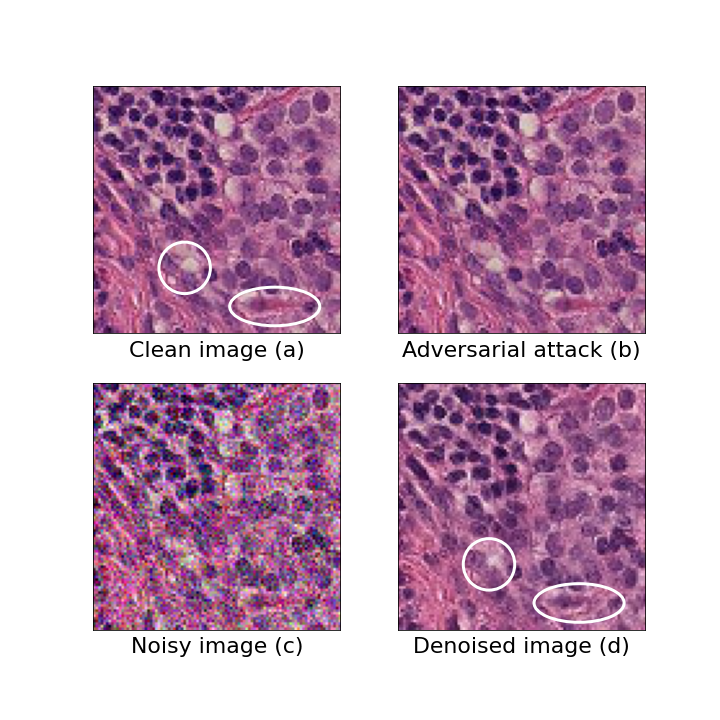
Denoising Diffusion Probabilistic Models as a Defense against Adversarial Attacks
Lars L Ankile, Anna Midgley, Sebastian Weisshaar
arXiv preprint, 2023
PDF
Exploration of Forecasting Paradigms and a Generalized Forecasting Framework
Lars L Ankile, Kjartan Krange
Master's thesis, NTNU, 2022
PDF •
Code
Deep Convolutional Neural Networks: A survey of the foundations, selected improvements, and some current applications
Lars L Ankile, Morgan Feet Heggland, Kjartan Krange
arXiv preprint, 2020
PDF
2024
Learning Fine and Dexterous Manipulation Workshop @ CoRL - Spotlight presentation on "From Imitation to Refinement"2024
Mastering Robotic Manipulation Workshop @ CoRL - Spotlight presentation on "Diffusion Policy Policy Optimization"2024
IROS Learning Track Spotlight - "JUICER: Data-Efficient Imitation Learning for Robotic Assembly"2023
Multi-Agent Security Workshop @ NeurIPS - "I See You!" oral presentation2023
Harvard MS Data Science Orientation Research Panel - Student Research Opportunities2022
NTNU PhD Course on Economic and Financial Forecasting - "Ensemble forecasting and the M4 competition"2025
Conference Reviewer - RSS'252024
High School Student Research Mentor - Advising projects on computer vision and robotics2023
Conference Reviewer - CoRL, ICML Workshops